案例:2009年英国国会议员开支丑闻#
MPs expenses scandal
data-journalism/data-journalism.github.io#54
import pandas as pd
import pylab as plt
plt.rcParams['font.sans-serif'] = ['Microsoft YaHei'] # 用来正常显示中文标签
plt.rcParams['axes.unicode_minus'] = False # 用来正常显示负号, 注意['SimHei']对应这句不行.
plt.style.use('ggplot')
ls './data/'
MPs' expenses claims, Jul-Dec, 2009.xlsx
data.js*
df = pd.read_excel("./data/MPs' expenses claims, Jul-Dec, 2009.xlsx")
df.head()
Name of member | Allowance Type | Expenditure Type | Date | Amount, £ | |
---|---|---|---|---|---|
0 | Adam Afriyie | Office running costs (IEP/AOE) | Incidentals | 2009-07-03 00:00:00 | 111.09 |
1 | Adam Afriyie | Office running costs (IEP/AOE) | Incidentals | 2009-11-05 00:00:00 | 111.09 |
2 | Adam Afriyie | Office running costs (IEP/AOE) | Incidentals | 2009-11-05 00:00:00 | 51.75 |
3 | Adam Holloway | Communications Expenditure | Delivery charges | 2009-11-10 00:00:00 | 258.22 |
4 | Adam Holloway | Communications Expenditure | Delivery charges | 2009-12-10 00:00:00 | 879.66 |
df.columns
Index(['Name of member', 'Allowance Type', 'Expenditure Type', 'Date',
'Amount, £'],
dtype='object')
len(df)
38925
df.describe()
Name of member | Allowance Type | Expenditure Type | Date | Amount, £ | |
---|---|---|---|---|---|
count | 38925 | 38925 | 38925 | 38925 | 38925 |
unique | 643 | 5 | 39 | 140 | 14441 |
top | Danny Alexander | Office running costs (IEP/AOE) | Incidentals | 2009-12-21 00:00:00 | 50 |
freq | 173 | 25787 | 8672 | 828 | 857 |
清洗数据#
df[df['Amount, £']=='Amount']
Name of member | Allowance Type | Expenditure Type | Date | Amount, £ | |
---|---|---|---|---|---|
27710 | Member Name | Allowance Type | Expenditure Type | Date | Amount |
df = df[df['Amount, £']!='Amount']
df[df['Name of member']=='John Randall']
Name of member | Allowance Type | Expenditure Type | Date | Amount, £ | |
---|---|---|---|---|---|
22221 | John Randall | Office running costs (IEP/AOE) | Accommodation costs | 2009-08-20 00:00:00 | -2134 |
22222 | John Randall | Office running costs (IEP/AOE) | Other equipment lease | 2009-07-01 00:00:00 | 25.08 |
df['Amount, £'] = [abs(float(i)) for i in df['Amount, £']]
描述数据#
df[['Name of member', 'Amount, £']].groupby("Name of member").agg('sum')
Amount, £ | |
---|---|
Name of member | |
Adam Afriyie | 273.93 |
Adam Holloway | 7415.06 |
Adam Ingram | 18578.90 |
Adam Price | 7874.73 |
Adrian Bailey | 10668.61 |
... | ... |
William Hague | 7370.98 |
William McCrea | 24689.34 |
William Rennie | 27257.33 |
Willie Bain | 822 |
Yvette Cooper | 21450.30 |
643 rows × 1 columns
dat1 = df[['Name of member', 'Amount, £']].groupby("Name of member").agg('sum')
dat1 = dat1.sort_values(by=['Amount, £'], ascending = False)
dat1[:10]
Amount, £ | |
---|---|
Name of member | |
Graham Allen | 42753.53 |
Michael Foster | 39999.69 |
Mark Hunter | 34779.11 |
Gordon Banks | 34471.90 |
Lynda Waltho | 34288.55 |
Joan Humble | 33395.89 |
Phil Wilson | 33031.23 |
Barbara Follett | 32861.14 |
Sian James | 32495.32 |
Helen Jones | 32209.45 |
dat1['Rank'] = range(1, len(dat1)+1)
dat1[:10]
Amount, £ | Rank | |
---|---|---|
Name of member | ||
Graham Allen | 42753.53 | 1 |
Michael Foster | 39999.69 | 2 |
Mark Hunter | 34779.11 | 3 |
Gordon Banks | 34471.90 | 4 |
Lynda Waltho | 34288.55 | 5 |
Joan Humble | 33395.89 | 6 |
Phil Wilson | 33031.23 | 7 |
Barbara Follett | 32861.14 | 8 |
Sian James | 32495.32 | 9 |
Helen Jones | 32209.45 | 10 |
plt.figure(figsize =(16, 6), dpi = 100)
plt.plot(dat1['Rank'], dat1['Amount, £'], 'ro')
# plt.yscale('log')
# plt.xscale('log')
plt.xlabel('Rank')
plt.ylabel('Amount, £')
plt.show()
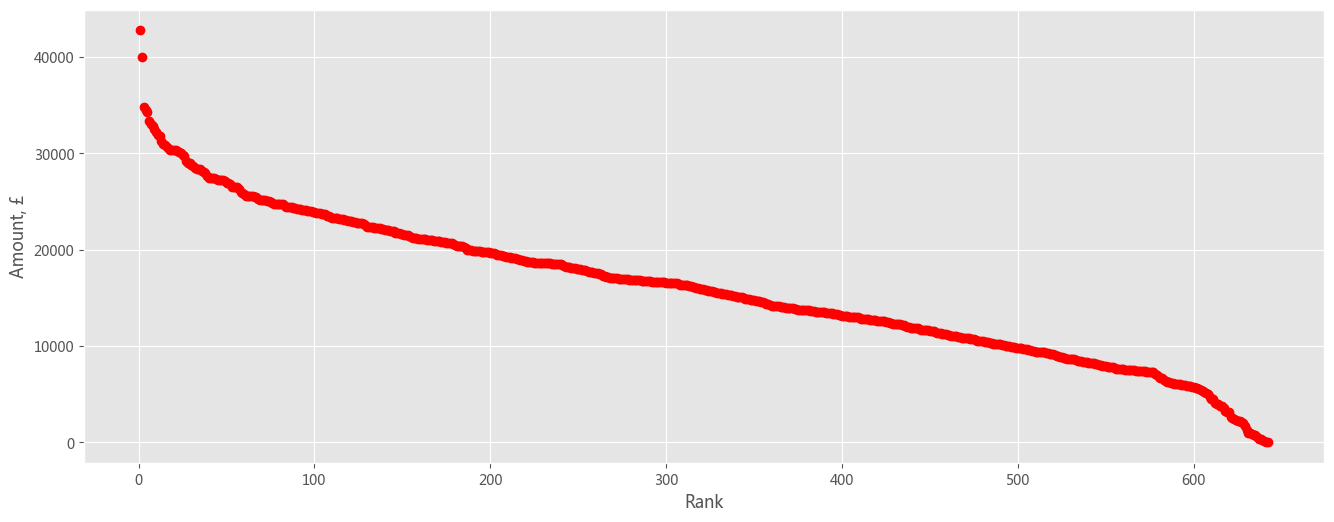
plt.figure(figsize =(16, 6), dpi = 100)
dat10 = dat1[:10]
plt.plot(dat10['Rank'], dat10['Amount, £'], 'ro')
# plt.yscale('log')
# plt.xscale('log')
plt.xlabel('Rank')
plt.ylabel('Amount, £')
plt.show()
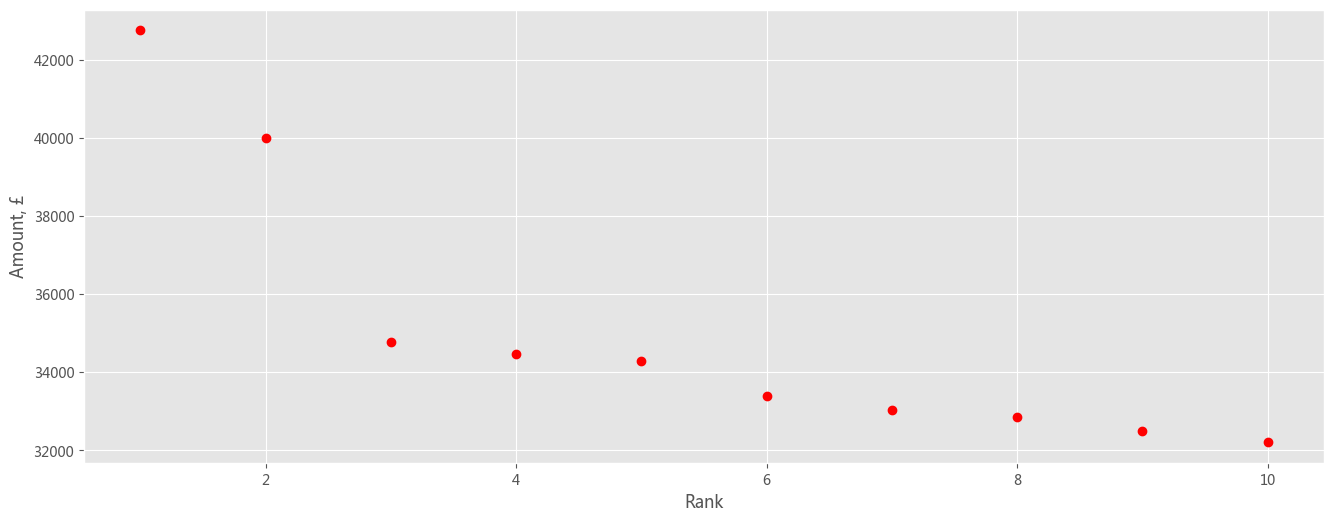
dat2 = df[['Allowance Type', 'Amount, £']].groupby("Allowance Type").agg('sum')
dat2 = dat2.sort_values(by=['Amount, £'], ascending = False)
dat2
Amount, £ | |
---|---|
Allowance Type | |
Office running costs (IEP/AOE) | 4561001.11 |
Cost of staying away from the main home (ACA/PAAE) | 3160432.72 |
Communications Expenditure | 2510511.02 |
Staffing non-payroll | 24414.17 |
dat3 = df[['Expenditure Type', 'Amount, £']].groupby("Expenditure Type").agg('sum')
dat3 = dat3.sort_values(by=['Amount, £'], ascending = False)
dat3
Amount, £ | |
---|---|
Expenditure Type | |
Accommodation costs | 1809184.32 |
Publications | 1097722.44 |
Mortgage interest | 1056568.92 |
Delivery charges | 1019891.50 |
Rent | 969231.40 |
Incidentals | 806222.41 |
Telephones | 677887.23 |
Other household costs | 463874.76 |
Subsistence | 373680.38 |
Advertising | 261207.10 |
Council tax | 226974.67 |
Agencies etc | 220883.47 |
Constituency Association payments | 187676.45 |
Utilities | 172737.37 |
Other equipment lease | 141508.52 |
Websites | 131689.98 |
IT equipment purchase | 117466.40 |
Professional fees | 90381.10 |
Other equipment purchase | 82802.68 |
Maintenance, janitorial | 79518.88 |
Hotel Expenses | 70102.59 |
Petty cash | 47442.51 |
Payments to individuals | 42585.55 |
Member travel (AOE) | 31841.92 |
Staff Subsistence | 24401.87 |
Staff office purchases | 22137.03 |
Staff travel rail | 8195.26 |
Staff telephone expenses | 6965.79 |
IT equipment lease | 4382.88 |
Staff subsistence | 4071.69 |
Staff travel car | 2888.88 |
Staff use of home expenses | 1969.68 |
Staff travel air | 800.41 |
Staff travel parking | 697.74 |
Staff travel taxi | 521.54 |
Staff travel public transport | 190.85 |
General Running Costs | 40.55 |
Staff travel tolls | 12.30 |
plt.figure(figsize =(16, 4), dpi = 100)
dat3['Amount, £'].plot(kind = 'bar')
plt.yscale('log')
plt.show()
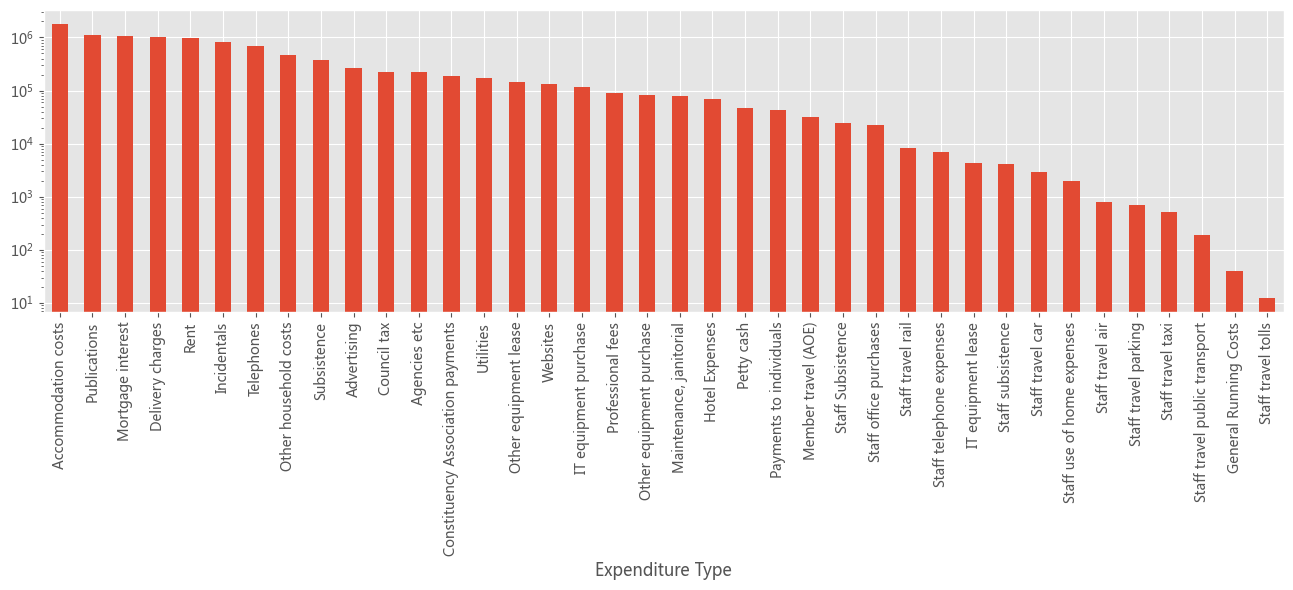
桑基图#
from pyecharts import options as opts
from pyecharts.charts import Sankey
nodes = [
{"name": "category1"},
{"name": "category2"},
{"name": "category3"},
{"name": "category4"},
{"name": "category5"},
{"name": "category6"},
]
links = [
{"source": "category1", "target": "category2", "value": 10},
{"source": "category2", "target": "category3", "value": 15},
{"source": "category3", "target": "category4", "value": 20},
{"source": "category5", "target": "category6", "value": 25},
]
c = (
Sankey()
.add(
"sankey",
nodes,
links,
linestyle_opt=opts.LineStyleOpts(opacity=0.2, curve=0.5, color="source"),
label_opts=opts.LabelOpts(position="right"),
)
.set_global_opts(title_opts=opts.TitleOpts(title="Sankey-基本示例"))
#.render("sankey_base.html")
.render_notebook()
)
c
dat4 = df[['Allowance Type','Expenditure Type', 'Amount, £']].groupby(['Expenditure Type', 'Allowance Type'], as_index = False).agg('sum')
dat4 = dat4.sort_values(by=['Amount, £'], ascending = False)
dat4
Expenditure Type | Allowance Type | Amount, £ | |
---|---|---|---|
0 | Accommodation costs | Office running costs (IEP/AOE) | 1809184.32 |
20 | Publications | Communications Expenditure | 1097722.44 |
13 | Mortgage interest | Cost of staying away from the main home (ACA/P... | 1056568.92 |
5 | Delivery charges | Communications Expenditure | 1019891.50 |
21 | Rent | Cost of staying away from the main home (ACA/P... | 969231.40 |
10 | Incidentals | Office running costs (IEP/AOE) | 806222.41 |
35 | Telephones | Office running costs (IEP/AOE) | 677887.23 |
16 | Other household costs | Cost of staying away from the main home (ACA/P... | 463874.76 |
34 | Subsistence | Cost of staying away from the main home (ACA/P... | 373680.38 |
1 | Advertising | Communications Expenditure | 261207.10 |
4 | Council tax | Cost of staying away from the main home (ACA/P... | 226974.67 |
2 | Agencies etc | Office running costs (IEP/AOE) | 220883.47 |
3 | Constituency Association payments | Office running costs (IEP/AOE) | 187676.45 |
36 | Utilities | Office running costs (IEP/AOE) | 172737.37 |
14 | Other equipment lease | Office running costs (IEP/AOE) | 141508.52 |
37 | Websites | Communications Expenditure | 131689.98 |
9 | IT equipment purchase | Office running costs (IEP/AOE) | 117466.40 |
19 | Professional fees | Office running costs (IEP/AOE) | 90381.10 |
15 | Other equipment purchase | Office running costs (IEP/AOE) | 82802.68 |
11 | Maintenance, janitorial | Office running costs (IEP/AOE) | 79518.88 |
7 | Hotel Expenses | Cost of staying away from the main home (ACA/P... | 70102.59 |
18 | Petty cash | Office running costs (IEP/AOE) | 47442.51 |
17 | Payments to individuals | Office running costs (IEP/AOE) | 42585.55 |
12 | Member travel (AOE) | Office running costs (IEP/AOE) | 31841.92 |
22 | Staff Subsistence | Staffing non-payroll | 24401.87 |
23 | Staff office purchases | Office running costs (IEP/AOE) | 22137.03 |
30 | Staff travel rail | Office running costs (IEP/AOE) | 8195.26 |
25 | Staff telephone expenses | Office running costs (IEP/AOE) | 6965.79 |
8 | IT equipment lease | Office running costs (IEP/AOE) | 4382.88 |
24 | Staff subsistence | Office running costs (IEP/AOE) | 4071.69 |
27 | Staff travel car | Office running costs (IEP/AOE) | 2888.88 |
33 | Staff use of home expenses | Office running costs (IEP/AOE) | 1969.68 |
26 | Staff travel air | Office running costs (IEP/AOE) | 800.41 |
28 | Staff travel parking | Office running costs (IEP/AOE) | 697.74 |
31 | Staff travel taxi | Office running costs (IEP/AOE) | 521.54 |
29 | Staff travel public transport | Office running costs (IEP/AOE) | 190.85 |
6 | General Running Costs | Office running costs (IEP/AOE) | 40.55 |
32 | Staff travel tolls | Staffing non-payroll | 12.30 |
nodes = dat4['Allowance Type'].unique().tolist() + dat4['Expenditure Type'].unique().tolist()
nodes = [{'name': i} for i in nodes]
links = [{'source': dat4['Allowance Type'][i], 'target': dat4['Expenditure Type'][i], 'value': dat4['Amount, £'][i]} for i in dat4.index]
from pyecharts import options as opts
from pyecharts.charts import Sankey
s = (
Sankey()
.add(
"sankey",
nodes,
links,
linestyle_opt=opts.LineStyleOpts(opacity=0.2, curve=0.5, color="source"),
label_opts=opts.LabelOpts(position="right"),
)
.set_global_opts(title_opts=opts.TitleOpts(title="Sankey Graph of UK MPS"))
)
s.render_notebook()
s.render("sankey_mps.html")
'/Users/datalab/github/data-journalism.github.io/notebook/sankey_mps.html'