第二章 数据科学的编程工具#
Python使用简介
王成军
人生苦短,我用Python。#
Python(/ˈpaɪθən/)是一种面向对象、解释型计算机程序设计语言
由Guido van Rossum于1989年底发明
第一个公开发行版发行于1991年
Python语法简洁而清晰
具有强大的标准库和丰富的第三方模块
它常被昵称为胶水语言
TIOBE编程语言排行榜“2010年度编程语言”
特点#
免费、功能强大、使用者众多
与R和MATLAB相比,Python是一门更易学、更严谨的程序设计语言。使用Python编写的脚本更易于理解和维护。
如同其它编程语言一样,Python语言的基础知识包括:类型、列表(list)和元组(tuple)、字典(dictionary)、条件、循环、异常处理等。
关于这些,初阶读者可以阅读《Beginning Python》一书(Hetland, 2005)。
Python中包含了丰富的类库。#
众多开源的科学计算软件包都提供了Python的调用接口,例如著名的计算机视觉库OpenCV。 Python本身的科学计算类库发展也十分完善,例如NumPy、SciPy和matplotlib等。 就社会网络分析而言,igraph, networkx, graph-tool, Snap.py等类库提供了丰富的网络分析工具
Python软件与IDE#
目前最新的Python版本为3.0,更稳定的2.7版本。 编译器是编写程序的重要工具。 免费的Python编译器有Spyder、PyCharm(免费社区版)、Ipython、Vim、 Emacs、 Eclipse(加上PyDev插件)。
Installing Anaconda Python#
Use the Anaconda Python
第三方包可以使用pip install的方法安装。#
可以点击ToolsOpen command prompt
然后在打开的命令窗口中输入:
pip install RISE
pip install RISE
NumPy /SciPy for scientific computing
pandas to make Python usable for data analysis
matplotlib to make graphics
scikit-learn for machine learning
pip install flownetwork
Requirement already satisfied: flownetwork in /opt/anaconda3/lib/python3.9/site-packages (3.1.0)
Requirement already satisfied: peppercorn in /opt/anaconda3/lib/python3.9/site-packages (from flownetwork) (0.6)
Note: you may need to restart the kernel to use updated packages.
from flownetwork import flownetwork as fn
import networkx as nx
import pylab as plt
import numpy as np
print(fn.__version__)
$version = py3.0.1$
help(fn.constructFlowNetwork)
Help on function constructFlowNetwork in module flownetwork.flownetwork:
constructFlowNetwork(C)
C is an array of two dimentions, e.g.,
C = np.array([[user1, item1],
[user1, item2],
[user2, item1],
[user2, item3]])
Return a balanced flow network
# constructing a flow network
demo = fn.attention_data
gd = fn.constructFlowNetwork(demo)
# drawing a demo network
fig = plt.figure(figsize=(12, 8),facecolor='white')
pos={0: np.array([ 0.2 , 0.8]),
2: np.array([ 0.2, 0.2]),
1: np.array([ 0.4, 0.6]),
6: np.array([ 0.4, 0.4]),
4: np.array([ 0.7, 0.8]),
5: np.array([ 0.7, 0.5]),
3: np.array([ 0.7, 0.2 ]),
'sink': np.array([ 1, 0.5]),
'source': np.array([ 0, 0.5])}
width=[float(d['weight']*1.2) for (u,v,d) in gd.edges(data=True)]
edge_labels=dict([((u,v,),d['weight']) for u,v,d in gd.edges(data=True)])
nx.draw_networkx_edge_labels(gd,pos,edge_labels=edge_labels, font_size = 15, alpha = .5)
nx.draw(gd, pos, node_size = 3000, node_color = 'orange',
alpha = 0.2, width = width, edge_color='orange',style='solid')
nx.draw_networkx_labels(gd,pos,font_size=18)
plt.show()
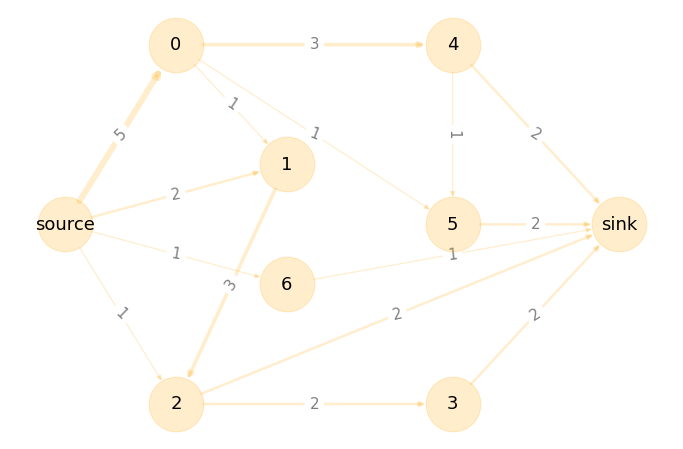
nx.info(gd)
'DiGraph with 9 nodes and 15 edges'
# flow matrix
m = fn.getFlowMatrix(gd)
m
matrix([[0., 5., 1., 0., 2., 1., 0., 0., 0.],
[0., 0., 0., 0., 1., 0., 0., 3., 1.],
[0., 0., 0., 1., 0., 0., 0., 0., 0.],
[0., 0., 0., 0., 0., 0., 0., 0., 0.],
[0., 0., 0., 0., 0., 3., 0., 0., 0.],
[0., 0., 0., 2., 0., 0., 2., 0., 0.],
[0., 0., 0., 2., 0., 0., 0., 0., 0.],
[0., 0., 0., 2., 0., 0., 0., 0., 1.],
[0., 0., 0., 2., 0., 0., 0., 0., 0.]])
fn.networkDissipate(gd)
defaultdict(<function flownetwork.flownetwork.networkDissipate.<locals>.<lambda>()>,
{0: [0, 5, 5],
1: [0, 3, 2],
2: [2, 4, 1],
6: [1, 1, 1],
3: [2, 2, 0],
4: [2, 3, 0],
5: [2, 2, 0]})
pip install --upgrade iching
Requirement already satisfied: iching in /opt/anaconda3/lib/python3.9/site-packages (3.7.2)
Note: you may need to restart the kernel to use updated packages.
import iching.iching as i
i.predict(200308030630, 202310271420)
Your birthday & your prediction time: 200308030630202310271420
there is a changing predict! Also run changePredict()
困 & 兑
本卦: 困卦原文困。亨,贞,大人吉,无咎。有言不信。象曰:泽无水,困。君子以致命遂志。白话文解释困卦:通泰。卜问王公贵族之事吉利,没有灾难。筮遇此爻,有罪之人无法申辩清楚。《象辞》说:本卦上卦为兑,兑为泽;下卦为坎,坎为水,水渗泽底,泽中干涸,是困卦的卦象。君子观此卦象,以处境艰难自励,穷且益坚,舍身捐命,以行其夙志。
《断易天机》解困卦兑上坎下,为兑宫初世卦。此卦君子受困于小人,阳为阴蔽,大人则吉而无咎。所闻之言没有诚信。
北宋易学家邵雍解泽上无水,受困穷之;万物不生,修德静守。得此卦者,陷入困境,事事不如意,宜坚守正道,等待时机。
台湾国学大儒傅佩荣解时运:身名皆困,不如安命。财运:财乏势危,不如归去。家宅:安全第一;女寡之象。身体:肾水已亏,险在眼前。
传统解卦这个卦是异卦(下坎上兑)相叠。兑为阴为泽喻悦;坎为阳为水喻险。泽水困,陷入困境,才智难以施展,仍坚守正道,自得其乐,必可成事,摆脱困境。大象:水在泽下,万物不生,喻君子困穷,小人滥盈之象。运势:诸事不如意,所谓龙游浅水遭虾戏。事业:境况十分不佳,遭受到很大的困难。人生面临巨大的考验,如采取不正当的手段,会愈陷愈深。相反,如身陷困逆境地而不失节操,自勉自坚,泰然处之,不失其志,终能成事。经商:面临激烈竞争,很有破产的可能。切勿失望,而应在困境中奋斗。为此,只能靠平日加强修养。认真反省自己的行为,总结教训,重新奋起,但也不宜浮躁,应缓慢而进。同时,更要警惕因致富发财,得意忘形而陷入新的困境。求名:欲速则不达。应以谦虚的态度,缓慢前进,应有坚定的志向,唯有志才能促成事业的成功。婚恋:以乐观态度冷静处理,尤应注重人品。决策:聪明智慧,但怀才不遇。若不因困境而失去信心,坚持努力上进,放弃侥幸心理,锲而不舍,虽不一定能守全实现自己的理想,但终会有所成。
台湾张铭仁解卦困:表示很大的困难被困住了,主大凶象,四大难卦第四卦。四处无援,最困难之时。事事很难再有进展,只好静待时机,是此时最好的选择。解释:被困住。特性:不满足感,不喜平淡生活,生活过于理想化,爱变化。自立自强,辛勤工作,善于用脑工作,不适合领导工作。运势:不如意,被小人欺,劳而无功,破损之灾。一事难成,运衰也。宜守己待时。家运:家庭之主有屈于下风,被内助压迫者,亦常生反弹,吵架滋事。为黑暗时期,宜忍辱负重,期待黎明到来。若不谨守正道者,有失和、破兆也。疾病:危重之象,注意口腔咽喉,泌尿系统,甚至性病。胎孕:胎安。将来劳碌命格。子女:劳苦之命,但行为端正者,终可得福也。周转:求人不如求己,凡事需量入为出。若为女色破财,当然求助无门。买卖:不能如愿,有挫折。等人:受到阻碍,不来或迟到。寻人:途中可遇,来者自来也。失物:不能寻回。外出:困难多,慎重考虑。考试:不理想。诉讼:凡事不宜过于执着,防牢狱之灾。求事:不得时亦不得意,再待时机。改行:不宜。开业:开业者须再待时。
初六爻辞初六。臀困于株木,入于幽谷,三岁不见。象曰:入于幽谷,幽不明也。白话文解释初六:臀部被狱吏的刑杖打伤,被投入黑暗的牢房中,三年不见其人。《象辞》说:进入了幽深的山谷,自然幽暗不明。
北宋易学家邵雍解凶:得此爻者,有惊忧,或有丧服之灾。做官的会退职。
台湾国学大儒傅佩荣解时运:渐入逆境,三年才转。财运:材木生意,运送不易。家宅:来往人少;男家卑微。身体:大凶之兆。
初六变卦初六爻动变得周易第58卦:兑为泽。这个卦是同卦(下泽上泽)相叠。泽为水。两泽相连,两水交流,上下相和,团结一致,朋友相助,欢欣喜悦。兑为悦也。同秉刚健之德,外抱柔和之姿,坚行正道,导民向上。
九二爻辞九二。困于洒食,朱绂方来,利用享祀。征凶,无咎。象曰:困于洒食,中有庆也。白话文解释九二:酒醉未醒,穿着红色服装的蛮夷前来进犯,忧患猝临,宜急祭神求佑。至于占问出征,则有危险。其他事无大的灾祸。《象辞》说:酒醉未醒,天予命赐公卿之服,因为九二之爻居下卦中位,这是将有喜庆之事的兆头。
北宋易学家邵雍解平:得此爻者,得贵人提携,营谋获利,静吉动凶。做官的有晋升之机。
台湾国学大儒傅佩荣解时运:有名有利,反为利用。财运:由商起家,往前则凶。家宅:富贵祭拜;婚姻即成。身体:饮食无度,收心祷告。
九二变卦九二爻动变得周易第45卦:泽地萃。这个卦是异卦(下坤上兑)相叠。坤为地、为顺;兑为泽、为水。泽泛滥淹没大地,人众多相互斗争,危机必四伏,务必顺天任贤,未雨绸缪,柔顺而又和悦,彼此相得益彰,安居乐业。萃,聚集、团结。
六三爻辞六三。困于石,据于疾藜。入于其宫,不见其妻,凶。象曰:据于疾藜,乘刚也;入于其宫,不见其妻,不祥也。白话文解释六三:被石头绊倒,被蒺藜刺伤,历难归家,妻子又不见了,这是凶险之兆。《象辞》说:被石头绊倒,被蒺藜刺伤,之所以屡遇艰难,因为六三阴爻居于九二阳爻之上,像弱者攀附于强暴之人,必受其挟持威凌。回到家中,妻子又不见了,这是不祥之兆。
北宋易学家邵雍解凶:得此爻者,多难之时,宜守正谨慎。
台湾国学大儒傅佩荣解时运:进退不得,身将不保。财运:财去命弱,下场堪虑。家宅:悼亡之屋。身体:无可救药。
六三变卦六三爻动变得周易第28卦:泽风大过。这个卦是异卦(下巽上兑)相叠。兑为泽、为悦,巽为木、为顺,泽水淹舟,遂成大错。阴阳爻相反,阳大阴小,行动非常,有过度形象,内刚外柔。
九四爻辞九四。来徐徐,困于金车,吝,有终。象曰:来徐徐,志在下也。虽不当位,有与也。白话文解释九四:其人被关押在囚车里,慢慢地走来。真不幸,但最后还是被释放。《象辞》说:行走缓慢,不求速进,志向卑微的表现。九四之爻居于九五之下,像人甘居下位,因为态度谦卑,倒能得人帮助。
北宋易学家邵雍解凶:得此爻者,谋事虽然不利,但终有出险之时,从商者或周转不利。做官的闲职者会被起用。
台湾国学大儒傅佩荣解时运:地位不当,受人所鄙。财运:货物失去,急救可保。家宅:慢些入住;事缓可成。身体:长期劳累,恐得归天。
九四变卦九四爻动变得周易第29卦:坎为水。这个卦是同卦(下坎上坎)相叠。坎为水、为险,两坎相重,险上加险,险阻重重。一阳陷二阴。所幸阴虚阳实,诚信可豁然贯通。虽险难重重,却方能显人性光彩。
九五爻辞九五。劓刖,困于赤绂。乃徐,有说,利用祭祀。象曰:劓刖,志未得也。乃徐有说,以中直也。利用祭祀,受福也。白话文解释九五:割了鼻子,断了腿,被身着红色服装的蛮夷虏去。后来慢慢找到脱身的机会,终于逃脱回家。宜急祭神酬谢。《象辞》说:割了鼻子,断了腿,是说其人不得志,身处险境。后来慢慢地脱离了险境,因为九五之爻居上卦中位,像人立身正直,自能化险为夷。宜祭祀鬼神,因为爻象指示:祈求鬼神保佑,承受其福荫。
北宋易学家邵雍解凶:得此爻者,先难后易,不良者有诉刑之扰,丧服之忧。做官的先阻后顺。
台湾国学大儒傅佩荣解时运:过刚必折,小心免祸。财运:货物清理,慢慢售出。家宅:鼻足之患;先疑后成。身体:头脚之病,调养祷告。
九五变卦九五爻动变得周易第40卦:雷水解。这个卦是异卦(下坎上震)相叠。震为雷、为动;坎为水、为险。险在内,动在外。严冬天地闭塞,静极而动。万象更新,冬去春来,一切消除,是为解。
上六爻辞上六。困于葛藟,于臲卼,曰动悔。有悔,征吉。象曰:困于葛藟,未当也。动悔有悔,吉行也。白话文解释上六:被葛藟绊倒,被小木桩刺伤,处境如此艰难,不宜有所行动,否则悔上加悔。至于占问出征则吉利。《象辞》说:被葛藟绊倒,因为行为不得当。悔悟到动则招悔,必能谦慎行事丽逢吉利。
北宋易学家邵雍解平:得此爻者,防惊忧丧服,惟商人、旅行者利有攸往。做官的会有刑罚束缚之忧。
台湾国学大儒傅佩荣解时运:厄运将终,收心努力。财运:久货可出,方可获利。家宅:修整旧宅;厘清瓜葛。身体:心神不安,迁地静养。
上六变卦上六爻动变得周易第6卦:天水讼。这个卦是异卦(下坎上乾)相叠。同需卦相反,互为“综卦”。乾为刚健,坎为险陷。刚与险,健与险,彼此反对,定生争讼。争讼非善事,务必慎重戒惧。
(O--__/\__--O)
(-------------(O---- |__|----O)----------------)
(-----------(O-----/-|__|-\------O)------------)
(-------(O-/_--_\-O)-------)
变卦: 兑卦原文兑。亨,利,贞。象曰:丽泽,兑。君子以朋友讲习。白话文解释兑卦:亨通。吉利的贞卜。《象辞》说:本卦为两兑相叠,兑为泽,两泽相连,两水交流是兑卦的卦象。君子观此卦象,从而广交朋友,讲习探索,推广见闻。
《断易天机》解兑卦兑上兑下,为兑宫本位卦。兑为喜悦、取悦,又为泽,泽中之水可以滋润万物,所占的人会很吉利。
北宋易学家邵雍解泽润万物,双重喜悦;和乐群伦,确守正道。得此卦者,多喜庆之事,人情和合,但应坚守正道,否则犯灾。
台湾国学大儒傅佩荣解时运:朋友支持,好好珍惜。财运:有人扶助,获利不难。家宅:友朋同住;因友成亲。身体:熟医可治。
传统解卦这个卦是同卦(下泽上泽)相叠。泽为水。两泽相连,两水交流,上下相和,团结一致,朋友相助,欢欣喜悦。兑为悦也。同秉刚健之德,外抱柔和之姿,坚行正道,导民向上。大象:两泽相依,更得泽中映月,美景良辰,令人怡悦。运势:悲喜交集,有誉有讥,守正道,诸事尚可称意。事业:由于善长人际关系,能团结他人,获得援助。因此,各项事业都十分顺利。只要本人坚持中正之道,动机纯正,是非分明,以诚心与人和悦,前途光明。经商:很有利,可以取得多种渠道的支持。但在顺利时切莫忘记谨慎小心的原则,尤其警惕上小人的当。求名:只要自己目的纯正,并有真才实学,一定可以受到多方面的热情帮助和资助,达到目的。婚恋:彼此满意,成功的可能性很大。但千万不要过于坚持己见。决策:为人聪颖,性格开朗,头脑灵活,心地善良,热心为公众服务,富有组织才能。因此,可以比较顺利地走上领导岗位。但一定要坚持中正原则,秉公办事,不得诌媚讨好上级,更不可欺压民众。永远保持谦虚品德,尤其不可过分自信,否则很容易为坏人包围。
台湾张铭仁解卦泽:表示少女纯真喜悦之象,却在纯真之中带有娇蛮、任性的态度。六冲卦象,大好大坏。忧喜参半!解释:喜悦,高兴。特性:细心,体贴,善解人意,口才佳,幽默感,宜从事公关,服务业。运势:有喜亦有忧,有誉亦有讥,虽得吉庆如意,然应守持正道,否则犯灾。家运:有和悦之气象,但要操守自律,行事不可越轨,有分寸可得吉运。若不操守自律,必犯色情之害而受殃。疾病:久病则凶,注意生活检点,戒酒色。胎孕:孕安。能带给家人喜悦,又与六亲和睦,有缘。但也不要过分溺爱才是。子女:骨肉情深,和好幸福之象。周转:可顺利,不须急也。买卖:有反覆之象,然尽力必成,可得大利之交易。等人:会来,且有喜讯相告。寻人:很快可知其下落。向西方寻可得。失物:遗失物似为金属或金钱,有望失而复得,但是迟一点。且多数已损毁或损失。外出:一路平安,即使遇到困难也会有人帮助,解脱困境。考试:成绩佳。诉讼:似为两个女性及金钱之事惹起,宜有和事佬出面调解。求事:得利,但亦不可太大意。改行:吉利。开业:吉利。
初九爻辞初九。和兑,吉。象曰:和兑之吉,行未疑也。白话文解释初九:和睦欢喜,吉利。《象辞》说:和睦欢喜之所以吉利,因为人际邦交无所猜疑。
北宋易学家邵雍解吉:得此爻者,人情和合,百谋皆遂。
台湾国学大儒傅佩荣解时运:以和为贵,诸事皆吉。财运:秋实可收,自然有利。家宅:和乐融融;室家得宜。身体:宽心无忧。
初九变卦初九爻动变得周易第47卦:泽水困。这个卦是异卦(下坎上兑)相叠。兑为阴为泽喻悦;坎为阳为水喻险。泽水困,陷入困境,才智难以施展,仍坚守正道,自得其乐,必可成事,摆脱困境。
九二爻辞九二。孚兑,吉,悔亡。象曰:孚兑之吉,信志也。白话文解释九二:优待俘虏,吉利,没有悔恨。《象辞》说:以诚信待人,人亦热忱待之,之所以吉利,因为互相之间有了信任。
北宋易学家邵雍解吉:得此爻者,正当好运,事事和顺。做官的有升迁之兆。
台湾国学大儒傅佩荣解时运:上下同心,自然吉祥。财运:以信为本,可长可远。家宅:与邻共富;阴阳相合。身体:疑病得解。
九二变卦九二爻动变得周易第17卦:泽雷随。这个卦是异卦(下震上兑)相叠,震为雷,为动;兑为悦,动而悦就是“随”。随指相互顺从,己有随物,物能随己,彼此沟通。随必依时顺势,有原则和条件,以坚贞为前提。
六三爻辞六三。来兑,凶。象曰:来兑之凶,位不当也白话文解释六三:以使人归服为乐,蕴藏着凶险。《象辞》说:以使人归服为乐,蕴藏着凶险,因为力小而任大,德薄而欲多,所行必不当。
北宋易学家邵雍解凶:得此爻者,会有意外之祸,甚者则失道忘身。做官的有听信谗言而遭辱之忧。
台湾国学大儒傅佩荣解时运:奔走营求,虽成亦辱。财运:无信之商,未来堪虑。家宅:去伪存诚;先合后离。身体:小心外祸。
六三变卦六三爻动变得周易第43卦:泽天夬。这个卦是异卦(下乾上兑)相叠。乾为天为健;兑为泽为悦。泽气上升,决注成雨,雨施大地,滋润万物。五阳去一阴,去之不难,决(去之意)即可,故名为夬(guài),夬即决。
九四爻辞九四。商兑,未宁,介疾有喜。象曰:九四之喜,有庆也。白话文解释九四:商谈恢复邦交之事,尚未达成协议,但两国的矛盾分歧有了愈合的趋势。《象辞》说:九四爻辞所讲的喜,即是指将有喜庆之事。
北宋易学家邵雍解平:得此爻者,从商获利,或进人口,不良者或有疾病,谋望不成。做官的会身居要职,升迁有望。
台湾国学大儒傅佩荣解时运:奋斗将成,斟酌行止。财运:忧心之事,商量解决。家宅:多疾不安;再三说媒而成。身体:心神不安,喜事舒怀。
九四变卦九四爻动变得周易第60卦:水泽节。这个卦是异卦(下兑上坎)相叠。兑为泽,坎为水。泽有水而流有限,多必溢于泽外。因此要有节度,故称节。节卦与涣卦相反,互为综卦,交相使用。天地有节度才能常新,国家有节度才能安稳,个人有节度才能完美。
九五爻辞九五。孚于剥,有厉。象曰:孚于剥,位正当也。白话文解释九五:被剥国俘虏。剥国无理挑衅,必遭惩罚(对我方而言,坏事将变为好事)。《象辞》说:当被侵剥之时,仍以诚信待人,正如九五阳爻所象,其人秉行中正之道,必能逢凶化吉。
北宋易学家邵雍解凶:得此爻者,时运不佳,多意外之祸。做官的会受到小人的诽谤。
台湾国学大儒傅佩荣解时运:居安思危,常得其昌。财运:虽有小损,信心仍在。家宅:诚信为上。身体:皮肤有疾,速治可愈。
九五变卦九五爻动变得周易第54卦:雷泽归妹。这个卦是异卦(下兑上震)相叠。震为动、为长男;兑为悦、为少女。以少女从长男,产生爱慕之情,有婚姻之动,有嫁女之象,故称归妹。
上六爻辞上六。引兑。象曰:上六引兑,未光也。白话文解释上六:引导大家和睦相处。《象辞》说:上六爻辞讲引导大家和睦相处,用意虽佳,但上六阴爻处一卦之尽头,像其人未必能一呼百应。
北宋易学家邵雍解平:得此爻者,营谋不顺,谨防有忧。
台湾国学大儒傅佩荣解时运:靠人扶持,平平之运。财运:有人指引,稍有小利。家宅:内忧外患;似非正聘。身体:化解内邪,才可保全。
上六变卦上六爻动变得周易第10卦:天泽履。这个卦是异卦(下兑上乾)相叠,乾为天,兑为泽,以天喻君,以泽喻民,原文:“履(踩)虎尾,不咥(咬)人”。因此,结果吉利。君上民下,各得其位。兑柔遇乾刚,所履危。履意为实践,卦义是脚踏实地的向前进取的意思。
import random, datetime
import numpy as np
import pylab as plt # plot
import matplotlib
import statsmodels.api as sm
from scipy.stats import norm
from scipy.stats.stats import pearsonr
#!pip install iching
with open('./data/the_republic_plato_gutenberg_pg1497.txt', 'r') as f:
lines = f.readlines()
len(lines)
24692
type(lines)
list
book = lines[8524:]
num = 0
for i in book:
if 'wrong' in i:
num+=1
print(num)
32
Variable Type#
# str, int, float, bool
type(False)
bool
type('Socrates')
str
# int
int('5')
5
# float
float(str(7.1))
#str(7.1)
7.1
range(10)
range(0, 10)
for i in range(1, 10+1):
print(i)
# range(1, 10)
1
2
3
4
5
6
7
8
9
10
dir & help#
当你想要了解对象的详细信息时使用
#dir(str)[-10:]
dir(str)
['__add__',
'__class__',
'__contains__',
'__delattr__',
'__dir__',
'__doc__',
'__eq__',
'__format__',
'__ge__',
'__getattribute__',
'__getitem__',
'__getnewargs__',
'__gt__',
'__hash__',
'__init__',
'__init_subclass__',
'__iter__',
'__le__',
'__len__',
'__lt__',
'__mod__',
'__mul__',
'__ne__',
'__new__',
'__reduce__',
'__reduce_ex__',
'__repr__',
'__rmod__',
'__rmul__',
'__setattr__',
'__sizeof__',
'__str__',
'__subclasshook__',
'capitalize',
'casefold',
'center',
'count',
'encode',
'endswith',
'expandtabs',
'find',
'format',
'format_map',
'index',
'isalnum',
'isalpha',
'isascii',
'isdecimal',
'isdigit',
'isidentifier',
'islower',
'isnumeric',
'isprintable',
'isspace',
'istitle',
'isupper',
'join',
'ljust',
'lower',
'lstrip',
'maketrans',
'partition',
'removeprefix',
'removesuffix',
'replace',
'rfind',
'rindex',
'rjust',
'rpartition',
'rsplit',
'rstrip',
'split',
'splitlines',
'startswith',
'strip',
'swapcase',
'title',
'translate',
'upper',
'zfill']
help(str)
Help on class str in module builtins:
class str(object)
| str(object='') -> str
| str(bytes_or_buffer[, encoding[, errors]]) -> str
|
| Create a new string object from the given object. If encoding or
| errors is specified, then the object must expose a data buffer
| that will be decoded using the given encoding and error handler.
| Otherwise, returns the result of object.__str__() (if defined)
| or repr(object).
| encoding defaults to sys.getdefaultencoding().
| errors defaults to 'strict'.
|
| Methods defined here:
|
| __add__(self, value, /)
| Return self+value.
|
| __contains__(self, key, /)
| Return key in self.
|
| __eq__(self, value, /)
| Return self==value.
|
| __format__(self, format_spec, /)
| Return a formatted version of the string as described by format_spec.
|
| __ge__(self, value, /)
| Return self>=value.
|
| __getattribute__(self, name, /)
| Return getattr(self, name).
|
| __getitem__(self, key, /)
| Return self[key].
|
| __getnewargs__(...)
|
| __gt__(self, value, /)
| Return self>value.
|
| __hash__(self, /)
| Return hash(self).
|
| __iter__(self, /)
| Implement iter(self).
|
| __le__(self, value, /)
| Return self<=value.
|
| __len__(self, /)
| Return len(self).
|
| __lt__(self, value, /)
| Return self<value.
|
| __mod__(self, value, /)
| Return self%value.
|
| __mul__(self, value, /)
| Return self*value.
|
| __ne__(self, value, /)
| Return self!=value.
|
| __repr__(self, /)
| Return repr(self).
|
| __rmod__(self, value, /)
| Return value%self.
|
| __rmul__(self, value, /)
| Return value*self.
|
| __sizeof__(self, /)
| Return the size of the string in memory, in bytes.
|
| __str__(self, /)
| Return str(self).
|
| capitalize(self, /)
| Return a capitalized version of the string.
|
| More specifically, make the first character have upper case and the rest lower
| case.
|
| casefold(self, /)
| Return a version of the string suitable for caseless comparisons.
|
| center(self, width, fillchar=' ', /)
| Return a centered string of length width.
|
| Padding is done using the specified fill character (default is a space).
|
| count(...)
| S.count(sub[, start[, end]]) -> int
|
| Return the number of non-overlapping occurrences of substring sub in
| string S[start:end]. Optional arguments start and end are
| interpreted as in slice notation.
|
| encode(self, /, encoding='utf-8', errors='strict')
| Encode the string using the codec registered for encoding.
|
| encoding
| The encoding in which to encode the string.
| errors
| The error handling scheme to use for encoding errors.
| The default is 'strict' meaning that encoding errors raise a
| UnicodeEncodeError. Other possible values are 'ignore', 'replace' and
| 'xmlcharrefreplace' as well as any other name registered with
| codecs.register_error that can handle UnicodeEncodeErrors.
|
| endswith(...)
| S.endswith(suffix[, start[, end]]) -> bool
|
| Return True if S ends with the specified suffix, False otherwise.
| With optional start, test S beginning at that position.
| With optional end, stop comparing S at that position.
| suffix can also be a tuple of strings to try.
|
| expandtabs(self, /, tabsize=8)
| Return a copy where all tab characters are expanded using spaces.
|
| If tabsize is not given, a tab size of 8 characters is assumed.
|
| find(...)
| S.find(sub[, start[, end]]) -> int
|
| Return the lowest index in S where substring sub is found,
| such that sub is contained within S[start:end]. Optional
| arguments start and end are interpreted as in slice notation.
|
| Return -1 on failure.
|
| format(...)
| S.format(*args, **kwargs) -> str
|
| Return a formatted version of S, using substitutions from args and kwargs.
| The substitutions are identified by braces ('{' and '}').
|
| format_map(...)
| S.format_map(mapping) -> str
|
| Return a formatted version of S, using substitutions from mapping.
| The substitutions are identified by braces ('{' and '}').
|
| index(...)
| S.index(sub[, start[, end]]) -> int
|
| Return the lowest index in S where substring sub is found,
| such that sub is contained within S[start:end]. Optional
| arguments start and end are interpreted as in slice notation.
|
| Raises ValueError when the substring is not found.
|
| isalnum(self, /)
| Return True if the string is an alpha-numeric string, False otherwise.
|
| A string is alpha-numeric if all characters in the string are alpha-numeric and
| there is at least one character in the string.
|
| isalpha(self, /)
| Return True if the string is an alphabetic string, False otherwise.
|
| A string is alphabetic if all characters in the string are alphabetic and there
| is at least one character in the string.
|
| isascii(self, /)
| Return True if all characters in the string are ASCII, False otherwise.
|
| ASCII characters have code points in the range U+0000-U+007F.
| Empty string is ASCII too.
|
| isdecimal(self, /)
| Return True if the string is a decimal string, False otherwise.
|
| A string is a decimal string if all characters in the string are decimal and
| there is at least one character in the string.
|
| isdigit(self, /)
| Return True if the string is a digit string, False otherwise.
|
| A string is a digit string if all characters in the string are digits and there
| is at least one character in the string.
|
| isidentifier(self, /)
| Return True if the string is a valid Python identifier, False otherwise.
|
| Call keyword.iskeyword(s) to test whether string s is a reserved identifier,
| such as "def" or "class".
|
| islower(self, /)
| Return True if the string is a lowercase string, False otherwise.
|
| A string is lowercase if all cased characters in the string are lowercase and
| there is at least one cased character in the string.
|
| isnumeric(self, /)
| Return True if the string is a numeric string, False otherwise.
|
| A string is numeric if all characters in the string are numeric and there is at
| least one character in the string.
|
| isprintable(self, /)
| Return True if the string is printable, False otherwise.
|
| A string is printable if all of its characters are considered printable in
| repr() or if it is empty.
|
| isspace(self, /)
| Return True if the string is a whitespace string, False otherwise.
|
| A string is whitespace if all characters in the string are whitespace and there
| is at least one character in the string.
|
| istitle(self, /)
| Return True if the string is a title-cased string, False otherwise.
|
| In a title-cased string, upper- and title-case characters may only
| follow uncased characters and lowercase characters only cased ones.
|
| isupper(self, /)
| Return True if the string is an uppercase string, False otherwise.
|
| A string is uppercase if all cased characters in the string are uppercase and
| there is at least one cased character in the string.
|
| join(self, iterable, /)
| Concatenate any number of strings.
|
| The string whose method is called is inserted in between each given string.
| The result is returned as a new string.
|
| Example: '.'.join(['ab', 'pq', 'rs']) -> 'ab.pq.rs'
|
| ljust(self, width, fillchar=' ', /)
| Return a left-justified string of length width.
|
| Padding is done using the specified fill character (default is a space).
|
| lower(self, /)
| Return a copy of the string converted to lowercase.
|
| lstrip(self, chars=None, /)
| Return a copy of the string with leading whitespace removed.
|
| If chars is given and not None, remove characters in chars instead.
|
| partition(self, sep, /)
| Partition the string into three parts using the given separator.
|
| This will search for the separator in the string. If the separator is found,
| returns a 3-tuple containing the part before the separator, the separator
| itself, and the part after it.
|
| If the separator is not found, returns a 3-tuple containing the original string
| and two empty strings.
|
| removeprefix(self, prefix, /)
| Return a str with the given prefix string removed if present.
|
| If the string starts with the prefix string, return string[len(prefix):].
| Otherwise, return a copy of the original string.
|
| removesuffix(self, suffix, /)
| Return a str with the given suffix string removed if present.
|
| If the string ends with the suffix string and that suffix is not empty,
| return string[:-len(suffix)]. Otherwise, return a copy of the original
| string.
|
| replace(self, old, new, count=-1, /)
| Return a copy with all occurrences of substring old replaced by new.
|
| count
| Maximum number of occurrences to replace.
| -1 (the default value) means replace all occurrences.
|
| If the optional argument count is given, only the first count occurrences are
| replaced.
|
| rfind(...)
| S.rfind(sub[, start[, end]]) -> int
|
| Return the highest index in S where substring sub is found,
| such that sub is contained within S[start:end]. Optional
| arguments start and end are interpreted as in slice notation.
|
| Return -1 on failure.
|
| rindex(...)
| S.rindex(sub[, start[, end]]) -> int
|
| Return the highest index in S where substring sub is found,
| such that sub is contained within S[start:end]. Optional
| arguments start and end are interpreted as in slice notation.
|
| Raises ValueError when the substring is not found.
|
| rjust(self, width, fillchar=' ', /)
| Return a right-justified string of length width.
|
| Padding is done using the specified fill character (default is a space).
|
| rpartition(self, sep, /)
| Partition the string into three parts using the given separator.
|
| This will search for the separator in the string, starting at the end. If
| the separator is found, returns a 3-tuple containing the part before the
| separator, the separator itself, and the part after it.
|
| If the separator is not found, returns a 3-tuple containing two empty strings
| and the original string.
|
| rsplit(self, /, sep=None, maxsplit=-1)
| Return a list of the words in the string, using sep as the delimiter string.
|
| sep
| The delimiter according which to split the string.
| None (the default value) means split according to any whitespace,
| and discard empty strings from the result.
| maxsplit
| Maximum number of splits to do.
| -1 (the default value) means no limit.
|
| Splits are done starting at the end of the string and working to the front.
|
| rstrip(self, chars=None, /)
| Return a copy of the string with trailing whitespace removed.
|
| If chars is given and not None, remove characters in chars instead.
|
| split(self, /, sep=None, maxsplit=-1)
| Return a list of the words in the string, using sep as the delimiter string.
|
| sep
| The delimiter according which to split the string.
| None (the default value) means split according to any whitespace,
| and discard empty strings from the result.
| maxsplit
| Maximum number of splits to do.
| -1 (the default value) means no limit.
|
| splitlines(self, /, keepends=False)
| Return a list of the lines in the string, breaking at line boundaries.
|
| Line breaks are not included in the resulting list unless keepends is given and
| true.
|
| startswith(...)
| S.startswith(prefix[, start[, end]]) -> bool
|
| Return True if S starts with the specified prefix, False otherwise.
| With optional start, test S beginning at that position.
| With optional end, stop comparing S at that position.
| prefix can also be a tuple of strings to try.
|
| strip(self, chars=None, /)
| Return a copy of the string with leading and trailing whitespace removed.
|
| If chars is given and not None, remove characters in chars instead.
|
| swapcase(self, /)
| Convert uppercase characters to lowercase and lowercase characters to uppercase.
|
| title(self, /)
| Return a version of the string where each word is titlecased.
|
| More specifically, words start with uppercased characters and all remaining
| cased characters have lower case.
|
| translate(self, table, /)
| Replace each character in the string using the given translation table.
|
| table
| Translation table, which must be a mapping of Unicode ordinals to
| Unicode ordinals, strings, or None.
|
| The table must implement lookup/indexing via __getitem__, for instance a
| dictionary or list. If this operation raises LookupError, the character is
| left untouched. Characters mapped to None are deleted.
|
| upper(self, /)
| Return a copy of the string converted to uppercase.
|
| zfill(self, width, /)
| Pad a numeric string with zeros on the left, to fill a field of the given width.
|
| The string is never truncated.
|
| ----------------------------------------------------------------------
| Static methods defined here:
|
| __new__(*args, **kwargs) from builtins.type
| Create and return a new object. See help(type) for accurate signature.
|
| maketrans(...)
| Return a translation table usable for str.translate().
|
| If there is only one argument, it must be a dictionary mapping Unicode
| ordinals (integers) or characters to Unicode ordinals, strings or None.
| Character keys will be then converted to ordinals.
| If there are two arguments, they must be strings of equal length, and
| in the resulting dictionary, each character in x will be mapped to the
| character at the same position in y. If there is a third argument, it
| must be a string, whose characters will be mapped to None in the result.
'cheng jun'.__add__(' is a big fan of Socrates!')
'cheng jun is a big fan of Socrates!'
#dir(str)[-10:]
' '.isspace()
True
'socrates the king'.__add__(' is the greatest.')
'socrates the king is the greatest.'
x = ' Hello WorlD '
dir(x)[-10:]
['rstrip',
'split',
'splitlines',
'startswith',
'strip',
'swapcase',
'title',
'translate',
'upper',
'zfill']
# lower
x.lower()
' hello world '
# upper
x.upper()
' HELLO WORLD '
# rstrip
x.rstrip()
' Hello WorlD'
# strip
x.strip()
'Hello WorlD'
# replace
x.replace('lo', 'l')
' Hell WorlD '
# split
# x.lower().strip().split(' ')
x.split('lo')
[' Hel', ' WorlD ']
# join
' - '.join(['a', '1'])
'a - 1'
type#
当你想要了解变量类型时使用type
x = 'hello world'
type(x)
#help(type(x))
str
Data Structure#
list, tuple, set, dictionary, array
l = [1,2,3,3] # list
t = (1, 2, 3, 3) # tuple
s = {1, 2, 3, 3} # set([1,2,3,3]) # set
d = {'a':1,'b':2,'c':3} # dict
a = np.array(l) # array
print(l, t, s, d, a)
[1, 2, 3, 3] (1, 2, 3, 3) {1, 2, 3} {'a': 1, 'b': 2, 'c': 3} [1 2 3 3]
l = [1,2,3,3] # list
l.append(4)
l
#help(list)
[1, 2, 3, 3, 4]
d = {'a':1,'b':2,'c':3} # dict
d.keys()
#help(dict)
dict_keys(['a', 'b', 'c'])
d = {'a':1,'b':2,'c':3} # dict
d.values()
dict_values([1, 2, 3])
d = {3:1,'b':3,'c':1} # dict
d['c']
1
d = {'a':1,'b':2,'c':3} # dict
d.items()
dict_items([('a', 1), ('b', 2), ('c', 3)])
定义函数#
def devidePlus(m, n): # 结尾是冒号
y = m/n + 1 # 注意:空格
return y # 注意:return
For 循环#
range(10)
range(0, 10)
range(1, 10)
range(1, 10)
for i in range(10):
print(i, i*10, i**2)
0 0 0
1 10 1
2 20 4
3 30 9
4 40 16
5 50 25
6 60 36
7 70 49
8 80 64
9 90 81
# for i in range(10):
# print(i*10)
for i in range(10):
print(devidePlus(i, 2))
1.0
1.5
2.0
2.5
3.0
3.5
4.0
4.5
5.0
5.5
# 列表内部的for循环(列表推演)
r = [devidePlus(i, 2) for i in range(10)]
r
[1.0, 1.5, 2.0, 2.5, 3.0, 3.5, 4.0, 4.5, 5.0, 5.5]
map函数#
def fahrenheit(T):
return (9/5)*T + 32
temp = [0, 22.5, 40,100]
#[fahrenheit(i) for i in temp]
F_temps = map(fahrenheit, temp)
print(*F_temps)
#[i for i in F_temps]
32.0 72.5 104.0 212.0
m1 = map(devidePlus, [4,3,2], [2, 1, 5])
print(*m1)
#print(*map(devidePlus, [4,3,2], [2, 1, 5]))
# 注意: 将(4, 2)作为一个组合进行计算,将(3, 1)作为一个组合进行计算
3.0 4.0 1.4
m2 = map(lambda x, y: x + y, [1, 3, 5, 7, 9], [2, 4, 6, 8, 10])
print(*m2)
3 7 11 15 19
m3 = map(lambda x, y, z: x + y - z, [1, 3, 5, 7, 9], [2, 4, 6, 8, 10], [3, 3, 2, 2, 5])
print(*m3)
0 4 9 13 14
if elif else#
j = 5.5
if j%2 == 1:
print(r'余数是1')
elif j%2 ==0:
print(r'余数是0')
else:
print(r'余数既不是1也不是0')
余数既不是1也不是0
x = 5
if x < 5:
y = -1
z = 5
elif x > 5:
y = 1
z = 11
else:
y = 0
z = 10
print(x, y, z)
5 0 10
while循环#
j = 0
while j <10:
print(j)
j+=1 # avoid dead loop
0
1
2
3
4
5
6
7
8
9
j = 0
while j <10:
if j%2 != 0:
print(j**2)
j+=1 # avoid dead loop
1
9
25
49
81
j = 0
while j <50:
if j == 30:
break
if j%2 != 0:
print(j**2)
j+=1 # avoid dead loop
1
9
25
49
81
121
169
225
289
361
441
529
625
729
841
a = 4
while a: # 0, None, False
print(a)
a -= 1
if a < 2:
a = {} # {}#[]#''#False #0 #None # []
4
3
2
try except#
def devidePlus(m, n): # 结尾是冒号
return m/n+ 1 # 注意:空格
error = []
for k, i in enumerate([2, 0, 5]):
# print(devidePlus(4, i))
try:
print(devidePlus(4, i))
except Exception as e:
#print(i, e)
error.append([k, i, e])
pass
error
3.0
1.8
[[1, 0, ZeroDivisionError('division by zero')]]
alist = [[1,1], [0, 0, 1]]
for i in alist:
try:
for j in i:
print(10 / j)
except Exception as e:
print(i, j, e)
pass
10.0
10.0
[0, 0, 1] 0 division by zero
alist = [[1,1], [0, 0, 1]]
for i in alist:
for j in i:
try:
print(10 / j)
except Exception as e:
print(j, e)
pass
10.0
10.0
0 division by zero
0 division by zero
10.0
Write and Read data#
data =[[i, i**2, i**3] for i in range(10)]
data
[[0, 0, 0],
[1, 1, 1],
[2, 4, 8],
[3, 9, 27],
[4, 16, 64],
[5, 25, 125],
[6, 36, 216],
[7, 49, 343],
[8, 64, 512],
[9, 81, 729]]
for i in data:
print('\t'.join([str(j) for j in i]))
#print('\t'.join(map(str, i)))
0 0 0
1 1 1
2 4 8
3 9 27
4 16 64
5 25 125
6 36 216
7 49 343
8 64 512
9 81 729
type(data)
list
len(data)
10
data[0]
[0, 0, 0]
help(f.write)
Help on built-in function write:
write(text, /) method of _io.TextIOWrapper instance
Write string to stream.
Returns the number of characters written (which is always equal to
the length of the string).
# 保存数据
data =[[i, i**2, i**3] for i in range(10000)]
f = open("data/data_write_to_file2023.txt", "w")
for i in data:
f.write('\t'.join([str(j) for j in i]) + '\n')
f.close()
with open('data/data_write_to_file2023.txt','r') as f:
data = f.readlines()
data[:5]
# print(data[0])
['0\t0\t0\n', '1\t1\t1\n', '2\t4\t8\n', '3\t9\t27\n', '4\t16\t64\n']
with open('./data/data_write_to_file1.txt','r') as f:
data = f.readlines(1000) #bytes 字节
len(data)
77
with open('./data/data_write_to_file1.txt','r') as f:
print(f.readline())
0 0 0
# f = [7, 2, 10, 4, 5]
# for k,i in enumerate(f):
# print(k,i)
with open('data/data_write_to_file1.txt', 'r') as f:
for k, i in enumerate(f):
if k%1000==0:
print(k, i)
0 0 0 0
1000 1000 1000000 1000000000
2000 2000 4000000 8000000000
3000 3000 9000000 27000000000
4000 4000 16000000 64000000000
5000 5000 25000000 125000000000
6000 6000 36000000 216000000000
7000 7000 49000000 343000000000
8000 8000 64000000 512000000000
9000 9000 81000000 729000000000
#from time import sleep
from tqdm import tqdm
from time import sleep
total = 0
with open('./data/data_write_to_file1.txt','r') as f:
for i in tqdm(f):
sleep(0.001)
total+=1
# for k, i in enumerate(f):
# if k % 1000 ==0:
# sleep(1)
# print(k, end = '\r')
print(total)
0000
with open('./data/data_write_to_file.txt','r') as f:
for k, i in enumerate(f):
if k%2000 == 0:
print(i)
0 0 0
2000 4000000 8000000000
4000 16000000 64000000000
6000 36000000 216000000000
8000 64000000 512000000000
data = []
line = '0\t0\t0\n'
line = line.replace('\n', '')
line = line.split('\t')
line = [int(i) for i in line] # convert str to int
data.append(line)
data
[[0, 0, 0]]
# 读取数据
data = []
with open('./data/data_write_to_file1.txt','r') as f:
for line in f:
line = line.replace('\n', '').split('\t')
line = [int(i) for i in line]
data.append(line)
#len(data)
data[-5:]
[[9995, 99900025, 998500749875],
[9996, 99920016, 998800479936],
[9997, 99940009, 999100269973],
[9998, 99960004, 999400119992],
[9999, 99980001, 999700029999]]
# 读取数据
data = []
with open('./data/data_write_to_file.txt','r') as f:
for line in f:
line = line.replace('\n', '').split('\t')
line = [int(i) for i in line]
data.append(line)
len(data)
10000
import pandas as pd
help(pd.read_csv)
Help on function read_csv in module pandas.io.parsers:
read_csv(filepath_or_buffer, sep=',', delimiter=None, header='infer', names=None, index_col=None, usecols=None, squeeze=False, prefix=None, mangle_dupe_cols=True, dtype=None, engine=None, converters=None, true_values=None, false_values=None, skipinitialspace=False, skiprows=None, skipfooter=None, nrows=None, na_values=None, keep_default_na=True, na_filter=True, verbose=False, skip_blank_lines=True, parse_dates=False, infer_datetime_format=False, keep_date_col=False, date_parser=None, dayfirst=False, iterator=False, chunksize=None, compression='infer', thousands=None, decimal='.', lineterminator=None, quotechar='"', quoting=0, escapechar=None, comment=None, encoding=None, dialect=None, tupleize_cols=False, error_bad_lines=True, warn_bad_lines=True, skip_footer=0, doublequote=True, delim_whitespace=False, as_recarray=False, compact_ints=False, use_unsigned=False, low_memory=True, buffer_lines=None, memory_map=False, float_precision=None)
Read CSV (comma-separated) file into DataFrame
Also supports optionally iterating or breaking of the file
into chunks.
Additional help can be found in the `online docs for IO Tools
<http://pandas.pydata.org/pandas-docs/stable/io.html>`_.
Parameters
----------
filepath_or_buffer : str, pathlib.Path, py._path.local.LocalPath or any object with a read() method (such as a file handle or StringIO)
The string could be a URL. Valid URL schemes include http, ftp, s3, and
file. For file URLs, a host is expected. For instance, a local file could
be file ://localhost/path/to/table.csv
sep : str, default ','
Delimiter to use. If sep is None, will try to automatically determine
this. Regular expressions are accepted and will force use of the python
parsing engine and will ignore quotes in the data.
delimiter : str, default None
Alternative argument name for sep.
header : int or list of ints, default 'infer'
Row number(s) to use as the column names, and the start of the data.
Default behavior is as if set to 0 if no ``names`` passed, otherwise
``None``. Explicitly pass ``header=0`` to be able to replace existing
names. The header can be a list of integers that specify row locations for
a multi-index on the columns e.g. [0,1,3]. Intervening rows that are not
specified will be skipped (e.g. 2 in this example is skipped). Note that
this parameter ignores commented lines and empty lines if
``skip_blank_lines=True``, so header=0 denotes the first line of data
rather than the first line of the file.
names : array-like, default None
List of column names to use. If file contains no header row, then you
should explicitly pass header=None
index_col : int or sequence or False, default None
Column to use as the row labels of the DataFrame. If a sequence is given, a
MultiIndex is used. If you have a malformed file with delimiters at the end
of each line, you might consider index_col=False to force pandas to _not_
use the first column as the index (row names)
usecols : array-like, default None
Return a subset of the columns.
Results in much faster parsing time and lower memory usage.
squeeze : boolean, default False
If the parsed data only contains one column then return a Series
prefix : str, default None
Prefix to add to column numbers when no header, e.g. 'X' for X0, X1, ...
mangle_dupe_cols : boolean, default True
Duplicate columns will be specified as 'X.0'...'X.N', rather than 'X'...'X'
dtype : Type name or dict of column -> type, default None
Data type for data or columns. E.g. {'a': np.float64, 'b': np.int32}
(Unsupported with engine='python'). Use `str` or `object` to preserve and
not interpret dtype.
engine : {'c', 'python'}, optional
Parser engine to use. The C engine is faster while the python engine is
currently more feature-complete.
converters : dict, default None
Dict of functions for converting values in certain columns. Keys can either
be integers or column labels
true_values : list, default None
Values to consider as True
false_values : list, default None
Values to consider as False
skipinitialspace : boolean, default False
Skip spaces after delimiter.
skiprows : list-like or integer, default None
Line numbers to skip (0-indexed) or number of lines to skip (int)
at the start of the file
skipfooter : int, default 0
Number of lines at bottom of file to skip (Unsupported with engine='c')
nrows : int, default None
Number of rows of file to read. Useful for reading pieces of large files
na_values : str or list-like or dict, default None
Additional strings to recognize as NA/NaN. If dict passed, specific
per-column NA values. By default the following values are interpreted as
NaN: `''`, `'#N/A'`, `'#N/A N/A'`, `'#NA'`, `'-1.#IND'`, `'-1.#QNAN'`, `'-NaN'`, `'-nan'`, `'1.#IND'`, `'1.#QNAN'`, `'N/A'`, `'NA'`, `'NULL'`, `'NaN'`, `'nan'`.
keep_default_na : bool, default True
If na_values are specified and keep_default_na is False the default NaN
values are overridden, otherwise they're appended to.
na_filter : boolean, default True
Detect missing value markers (empty strings and the value of na_values). In
data without any NAs, passing na_filter=False can improve the performance
of reading a large file
verbose : boolean, default False
Indicate number of NA values placed in non-numeric columns
skip_blank_lines : boolean, default True
If True, skip over blank lines rather than interpreting as NaN values
parse_dates : boolean or list of ints or names or list of lists or dict, default False
* boolean. If True -> try parsing the index.
* list of ints or names. e.g. If [1, 2, 3] -> try parsing columns 1, 2, 3
each as a separate date column.
* list of lists. e.g. If [[1, 3]] -> combine columns 1 and 3 and parse as
a single date column.
* dict, e.g. {'foo' : [1, 3]} -> parse columns 1, 3 as date and call result
'foo'
Note: A fast-path exists for iso8601-formatted dates.
infer_datetime_format : boolean, default False
If True and parse_dates is enabled for a column, attempt to infer
the datetime format to speed up the processing
keep_date_col : boolean, default False
If True and parse_dates specifies combining multiple columns then
keep the original columns.
date_parser : function, default None
Function to use for converting a sequence of string columns to an array of
datetime instances. The default uses ``dateutil.parser.parser`` to do the
conversion. Pandas will try to call date_parser in three different ways,
advancing to the next if an exception occurs: 1) Pass one or more arrays
(as defined by parse_dates) as arguments; 2) concatenate (row-wise) the
string values from the columns defined by parse_dates into a single array
and pass that; and 3) call date_parser once for each row using one or more
strings (corresponding to the columns defined by parse_dates) as arguments.
dayfirst : boolean, default False
DD/MM format dates, international and European format
iterator : boolean, default False
Return TextFileReader object for iteration or getting chunks with
``get_chunk()``.
chunksize : int, default None
Return TextFileReader object for iteration. `See IO Tools docs for more
information
<http://pandas.pydata.org/pandas-docs/stable/io.html#io-chunking>`_ on
``iterator`` and ``chunksize``.
compression : {'infer', 'gzip', 'bz2', None}, default 'infer'
For on-the-fly decompression of on-disk data. If 'infer', then use gzip or
bz2 if filepath_or_buffer is a string ending in '.gz' or '.bz2',
respectively, and no decompression otherwise. Set to None for no
decompression.
thousands : str, default None
Thousands separator
decimal : str, default '.'
Character to recognize as decimal point (e.g. use ',' for European data).
lineterminator : str (length 1), default None
Character to break file into lines. Only valid with C parser.
quotechar : str (length 1), optional
The character used to denote the start and end of a quoted item. Quoted
items can include the delimiter and it will be ignored.
quoting : int or csv.QUOTE_* instance, default None
Control field quoting behavior per ``csv.QUOTE_*`` constants. Use one of
QUOTE_MINIMAL (0), QUOTE_ALL (1), QUOTE_NONNUMERIC (2) or QUOTE_NONE (3).
Default (None) results in QUOTE_MINIMAL behavior.
escapechar : str (length 1), default None
One-character string used to escape delimiter when quoting is QUOTE_NONE.
comment : str, default None
Indicates remainder of line should not be parsed. If found at the beginning
of a line, the line will be ignored altogether. This parameter must be a
single character. Like empty lines (as long as ``skip_blank_lines=True``),
fully commented lines are ignored by the parameter `header` but not by
`skiprows`. For example, if comment='#', parsing '#empty\na,b,c\n1,2,3'
with `header=0` will result in 'a,b,c' being
treated as the header.
encoding : str, default None
Encoding to use for UTF when reading/writing (ex. 'utf-8'). `List of Python
standard encodings
<https://docs.python.org/3/library/codecs.html#standard-encodings>`_
dialect : str or csv.Dialect instance, default None
If None defaults to Excel dialect. Ignored if sep longer than 1 char
See csv.Dialect documentation for more details
tupleize_cols : boolean, default False
Leave a list of tuples on columns as is (default is to convert to
a Multi Index on the columns)
error_bad_lines : boolean, default True
Lines with too many fields (e.g. a csv line with too many commas) will by
default cause an exception to be raised, and no DataFrame will be returned.
If False, then these "bad lines" will dropped from the DataFrame that is
returned. (Only valid with C parser)
warn_bad_lines : boolean, default True
If error_bad_lines is False, and warn_bad_lines is True, a warning for each
"bad line" will be output. (Only valid with C parser).
Returns
-------
result : DataFrame or TextParser
df = pd.read_csv('./data/data_write_to_file2023.txt',
sep = '\t', names = ['a', 'b', 'c'])
df.tail()
#len(df)
a | b | c | |
---|---|---|---|
9995 | 9995 | 99900025 | 998500749875 |
9996 | 9996 | 99920016 | 998800479936 |
9997 | 9997 | 99940009 | 999100269973 |
9998 | 9998 | 99960004 | 999400119992 |
9999 | 9999 | 99980001 | 999700029999 |
保存中间步骤产生的字典数据#
import json
data_dict = {'a':1, 'b':2, 'c':3}
with open('./data/save_dict.json', 'w') as f:
json.dump(data_dict, f)
import json
dd = json.load(open("./data/save_dict.json"))
dd
{'a': 1, 'b': 2, 'c': 3}
重新读入json#
保存中间步骤产生的列表数据#
data_list = list(range(10))
with open('./data/save_list.json', 'w') as f:
json.dump(data_list, f)
dl = json.load(open("./data/save_list.json"))
dl
[0, 1, 2, 3, 4, 5, 6, 7, 8, 9]
使用matplotlib绘图#
#
%matplotlib inline
import matplotlib.pyplot as plt
x = range(1, 100)
y = [i**-3 for i in x]
plt.plot(x, y, 'b-s')
plt.ylabel('$p(k)$', fontsize = 20)
plt.xlabel('$k$', fontsize = 20)
plt.xscale('log')
plt.yscale('log')
plt.title('Degree Distribution')
plt.show()
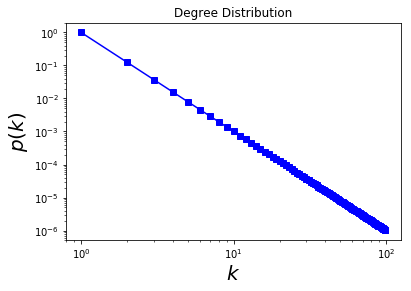
import numpy as np
# red dashes, blue squares and green triangles
t = np.arange(0., 5., 0.2)
plt.plot(t, t, 'r--')
plt.plot(t, t**2, 'bs')
plt.plot(t, t**3, 'g^')
plt.show()
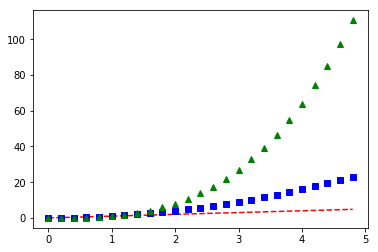
# red dashes, blue squares and green triangles
t = np.arange(0., 5., 0.2)
plt.plot(t, t**2, 'b-s', label = '1')
plt.plot(t, t**2.5, 'r-o', label = '2')
plt.plot(t, t**3, 'g-^', label = '3')
plt.annotate(r'$\alpha = 3$', xy=(3.5, 40), xytext=(2, 80),
arrowprops=dict(facecolor='black', shrink=0.05),
fontsize = 20)
plt.ylabel('$f(t)$', fontsize = 20)
plt.xlabel('$t$', fontsize = 20)
plt.legend(loc=2,numpoints=1,fontsize=10)
plt.show()
# plt.savefig('/Users/chengjun/GitHub/cjc/figure/save_figure.png',
# dpi = 300, bbox_inches="tight",transparent = True)
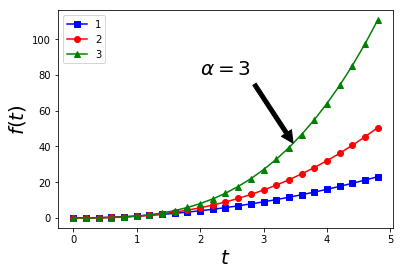
import matplotlib.pyplot as plt
import seaborn as sns
plt.figure(figsize=(5,5))
sns.set(style="whitegrid")
plt.figure(1)
plt.subplot(221)
plt.plot(t, t, 'r--')
plt.text(2, 0.8*np.max(t), r'$\alpha = 1$', fontsize = 20)
plt.subplot(222)
plt.plot(t, t**2, 'bs')
plt.text(2, 0.8*np.max(t**2), r'$\alpha = 2$', fontsize = 20)
plt.subplot(223)
plt.plot(t, t**3, 'g^')
plt.text(2, 0.8*np.max(t**3), r'$\alpha = 3$', fontsize = 20)
plt.subplot(224)
plt.plot(t, t**4, 'r-o')
plt.text(2, 0.8*np.max(t**4), r'$\alpha = 4$', fontsize = 20)
plt.show()
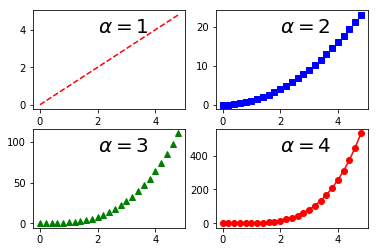
def f(t):
return np.exp(-t) * np.cos(2*np.pi*t)
t1 = np.arange(0.0, 5.0, 0.1)
t2 = np.arange(0.0, 5.0, 0.02)
plt.figure(1)
plt.subplot(211)
plt.plot(t1, f(t1), 'bo')
plt.plot(t2, f(t2), 'k')
plt.subplot(212)
plt.plot(t2, np.cos(2*np.pi*t2), 'r--')
plt.show()
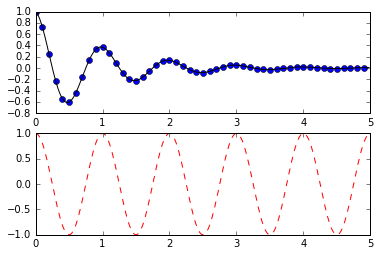
import matplotlib.gridspec as gridspec
import numpy as np
t = np.arange(0., 5., 0.2)
gs = gridspec.GridSpec(3, 3)
ax1 = plt.subplot(gs[0, :])
plt.plot(t, t**2, 'b-s')
ax2 = plt.subplot(gs[1,:-1])
plt.plot(t, t**2, 'g-s')
ax3 = plt.subplot(gs[1:, -1])
plt.plot(t, t**2, 'r-o')
ax4 = plt.subplot(gs[-1,0])
plt.plot(t, t**2, 'g-^')
ax5 = plt.subplot(gs[-1,1])
plt.plot(t, t**2, 'b-<')
plt.tight_layout()
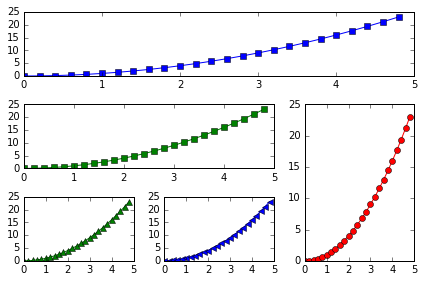
def OLSRegressPlot(x,y,col,xlab,ylab):
xx = sm.add_constant(x, prepend=True)
res = sm.OLS(y,xx).fit()
constant, beta = res.params
r2 = res.rsquared
lab = r'$\beta = %.2f, \,R^2 = %.2f$' %(beta,r2)
plt.scatter(x,y,s=60,facecolors='none', edgecolors=col)
plt.plot(x,constant + x*beta,"red",label=lab)
plt.legend(loc = 'upper left',fontsize=16)
plt.xlabel(xlab,fontsize=26)
plt.ylabel(ylab,fontsize=26)
x = np.random.randn(50)
y = np.random.randn(50) + 3*x
pearsonr(x, y)
fig = plt.figure(figsize=(10, 4),facecolor='white')
OLSRegressPlot(x,y,'RoyalBlue',r'$x$',r'$y$')
plt.show()
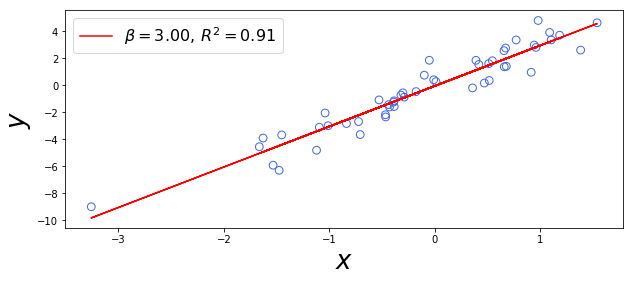
fig = plt.figure(figsize=(7, 4),facecolor='white')
data = norm.rvs(10.0, 2.5, size=5000)
mu, std = norm.fit(data)
plt.hist(data, bins=25, normed=True, alpha=0.6, color='g')
xmin, xmax = plt.xlim()
x = np.linspace(xmin, xmax, 100)
p = norm.pdf(x, mu, std)
plt.plot(x, p, 'r', linewidth=2)
title = r"$\mu = %.2f, \, \sigma = %.2f$" % (mu, std)
plt.title(title,size=16)
plt.show()
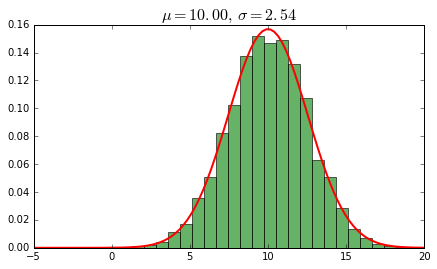
import pandas as pd
df = pd.read_csv('../data/data_write_to_file.txt', sep = '\t', names = ['a', 'b', 'c'])
df[:5]
a | b | c | |
---|---|---|---|
0 | 0 | 0 | 0 |
1 | 1 | 1 | 1 |
2 | 2 | 4 | 8 |
3 | 3 | 9 | 27 |
4 | 4 | 16 | 64 |
df.plot.line()
plt.yscale('log')
plt.ylabel('$values$', fontsize = 20)
plt.xlabel('$index$', fontsize = 20)
plt.show()
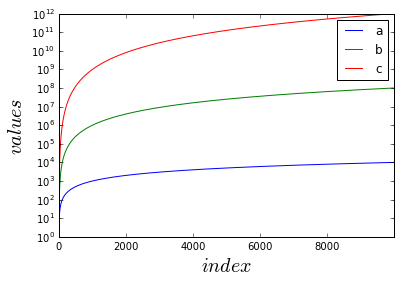
df.plot.scatter(x='a', y='b')
plt.show()
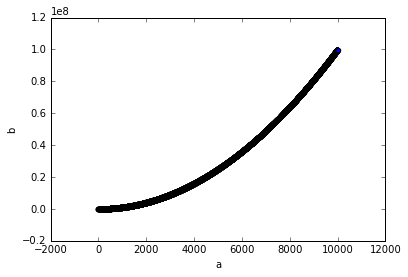
df.plot.hexbin(x='a', y='b', gridsize=25)
plt.show()
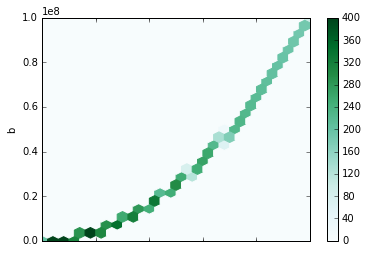
df['a'].plot.kde()
plt.show()
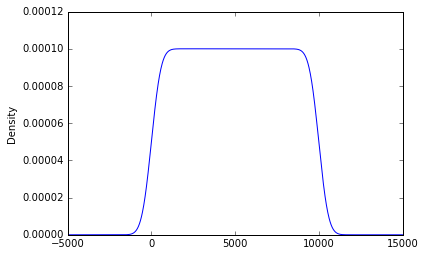
bp = df.boxplot()
plt.yscale('log')
plt.show()
/Users/chengjun/anaconda/lib/python2.7/site-packages/ipykernel/__main__.py:1: FutureWarning:
The default value for 'return_type' will change to 'axes' in a future release.
To use the future behavior now, set return_type='axes'.
To keep the previous behavior and silence this warning, set return_type='dict'.
if __name__ == '__main__':
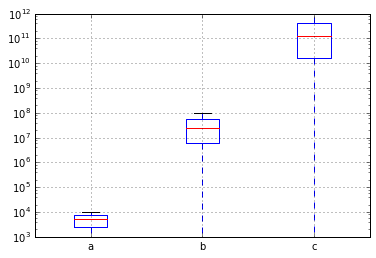
df['c'].diff().hist()
plt.show()
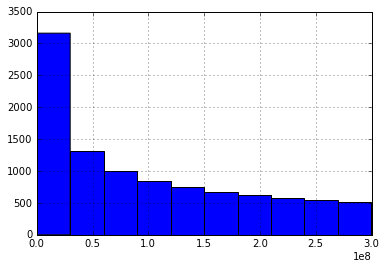
df.plot.hist(stacked=True, bins=20)
# plt.yscale('log')
plt.show()
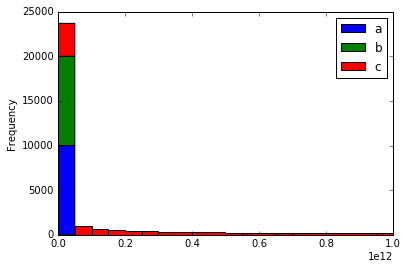
To be a programmer is to develop a carefully managed relationship with error. There’s no getting around it. You either make your accommodations with failure, or the work will become intolerable.
Ellen Ullman (an American computer programmer and author)
This is the end.#
Thank you for your attention.